Use of AI software in recruitment raises questions about accuracy, bias
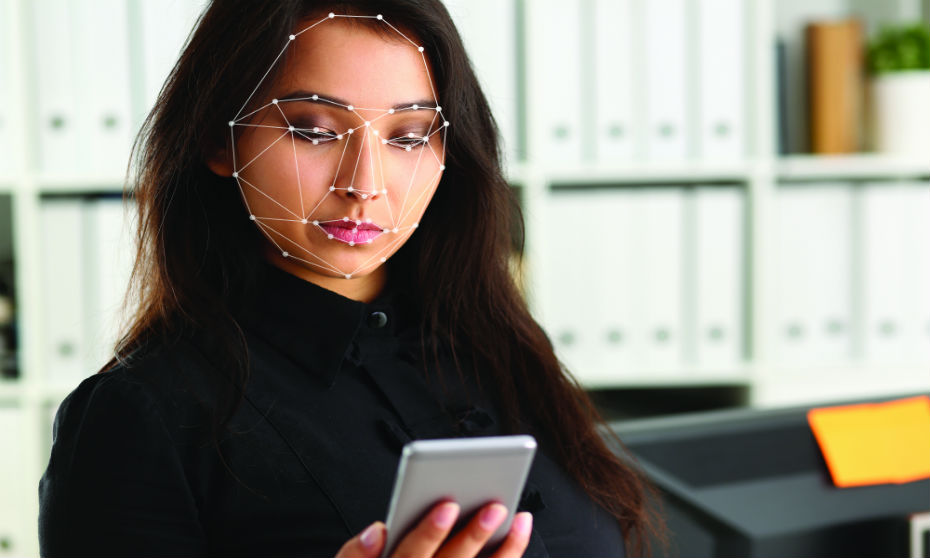
Just over a year ago, Amazon found itself uncomfortably in the spotlight when it abandoned an artificial intelligence (AI) recruitment tool that turned out to be biased against women because the system had basically taught itself that male candidates were preferable.